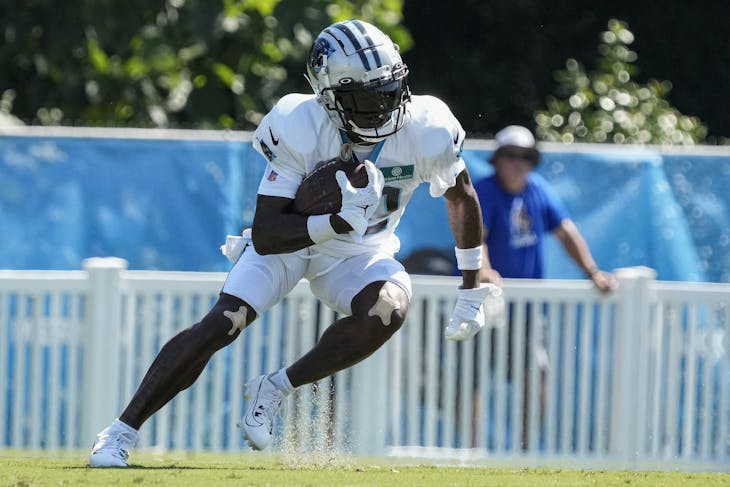
Welcome to Regression Alert, your weekly guide to using regression to predict the future with uncanny accuracy.
For those who are new to the feature, here's the deal: every week, I dive into the topic of regression to the mean. Sometimes, I'll explain what it really is, why you hear so much about it, and how you can harness its power for yourself. Sometimes, I'll give some practical examples of regression at work.
In weeks where I'm giving practical examples, I will select a metric to focus on. I'll rank all players in the league according to that metric and separate the top players into Group A and the bottom players into Group B. I will verify that the players in Group A have outscored the players in Group B to that point in the season. And then I will predict that, by the magic of regression, Group B will outscore Group A going forward.
Crucially, I don't get to pick my samples (other than choosing which metric to focus on). If I'm looking at receivers and Justin Jefferson is one of the top performers in my sample, then Justin Jefferson goes into Group A, and may the fantasy gods show mercy on my predictions.
Most importantly, because predictions mean nothing without accountability, I report on all my results in real time and end each season with a summary. Here's a recap from last year detailing every prediction I made in 2022, along with all results from this column's six-year history (my predictions have gone 36-10, a 78% success rate). And here are similar roundups from 2021, 2020, 2019, 2018, and 2017.
The Scorecard
In Week 2, I broke down what regression to the mean really is, what causes it, how we can benefit from it, and what the guiding philosophy of this column would be. No specific prediction was made.
In Week 3, I dove into the reasons why yards per carry is almost entirely noise, shared some research to that effect, and predicted that the sample of backs with lots of carries but a poor per-carry average would outrush the sample with fewer carries but more yards per carry.
In Week 4, I explained that touchdowns follow yards, but yards don't follow touchdowns, and predicted that high-yardage, low-touchdown receivers were going to start scoring a lot more going forward.
In Week 5, we revisited one of my favorite findings. We know that early-season overperformers and early-season underperformers tend to regress, but every year, I test the data and confirm that preseason ADP is still as predictive as early-season results even through four weeks of the season. I sliced the sample in several new ways to see if we could find some split where early-season performance was more predictive than ADP, but I failed in all instances.
In Week 6, I talked about how when we're confronted with an unfamiliar statistic, checking the leaderboard can be a quick and easy way to guess how prone that statistic will be to regression.
In Week 7, I discussed how just because something is an outlier doesn't mean it's destined to regress and predicted that this season's passing yardage per game total would remain significantly below recent levels.
In Week 8, I wrote about why statistics for quarterbacks don't tend to regress as much as statistics for receivers or running backs and why interception rate was the one big exception. I predicted that low-interception teams would start throwing more picks than high-interception teams going forward.
In Week 9, I explained the critical difference between regression to the mean (the tendency for players whose performance had deviated from their underlying average to return to that average) and the gambler's fallacy (the belief that players who deviate in one direction are "due" to deviate in the opposite direction to offset).
In Week 10, I discussed not only finding stats that were likely to regress to their "true mean", but also how we could estimate what that true mean might be.
In Week 11, I explained why larger samples work to regression's benefit and made another yards per carry prediction.
In Week 12, I used a simple model to demonstrate why outlier performances typically require a player to be both lucky and good.
In Week 13, I talked about how a player's mean wasn't a fixed target and predicted that rookie performance would improve later in the season.
In Week 14, I mentioned that hot and cold streaks are mostly a mirage and that all players tend to regress strongly toward their full-season averages.
Statistic Being Tracked | Performance Before Prediction | Performance Since Prediction | Remaining Weeks |
---|---|---|---|
Yards Per Carry | Group A had 42% more rushing yards/game | Group A has 10% more rushing yards/game | None (Loss) |
Yard-to-TD Ratio | Group A had 7% more points/game | Group B has 38% more points/game | None (Win) |
Passing Yards | Teams averaged 218.4 yards/game | Teams average 219.8 yards/game | 3 |
Interceptions Thrown | Group A threw 25% fewer interceptions | Group B has thrown 11% fewer interceptions | None (Win) |
Yards Per Carry | Group A had 10% more rushing yards/game | Group A has 19% more rushing yards/game | None (Loss) |
Rookie PPG | Group A averaged 9.05 ppg | Group A averages 9.04 ppg | 2 |
Rookie Improvement | 62% are beating their prior average | 2 | |
"Hot" Players Regress | Players were performing at an elevated level | Players have regressed 91% to season avg. | 3 |
I wrote last week about how both Group A and Group B had an identical yards per carry advantage since our prediction. Well... so much for that-- Group A averaged 5.57 yards per carry in Week 14 while Group B averaged 3.17, the highest and lowest averages we've seen in any week in either of our two failed yards per carry predictions, ending any hope of a last-minute comeback.
Group A's yards per carry did regress overall, falling from 5.05 to 4.40, which is around league average. But Group B's ypc remained low, and its workload cratered. While our first ypc prediction saw the groups regress (but not enough to cover the initial aggressive prediction), this time Group A saw its lead widen from where it was at when the prediction was made. Just a cursed year overall for yards per carry.
Our rookie prediction is faring better so far. The points per game hasn't moved, but that's an artifact of the Tank Dell injury-- excluding his performance prior to the prediction and his zero in Week 13, rookie scoring is up 10%. More importantly, 62% of rookies have seen their scoring rise. It should continue to rise higher still over the next two weeks.
Some of our best predictions have come up short this year, but our "hot players regress" prediction doesn't look like it'll join them. In the first week of action, we saw the hottest players in fantasy football regress nearly 100% of the way back to their full-season average.
Your Best Team Is Probably Going To Lose
My s*** doesn't work in the playoffs. My job is to get us to the playoffs. What happens after that is f****** luck.
-Billy Beane
We spend all year working to get our teams into the playoffs. We seek out every edge we can exploit. We learn about regression to the mean, and we harness the forces of randomness to carry us onward.
But randomness does not hold a harness well. It is wild, and it is chaotic. And so, despite our efforts, our most likely reward for reaching the postseason is a season-ending loss.
It's important to realize that this is not a failure on our part. It's tempting to think that we can control the chaos; we can find the exact right sleeper against the exact right matchup and push ourselves over the finish line. Or maybe we're focused on the order and not the chaos. Maybe most teams are more likely than not to lose, but certainly not our best ones. Certainly, our 12-2 team, armed with a bye and outscoring all competition by 10 points per game, has at least a better-than-a-coinflip shot at the title.
That's simply not the case. Sure, someone is going to end the season holding a trophy. But for any given team, it's more likely than not that someone isn't you.
Continue reading this content with a PRO subscription.
"Footballguys is the best premium
fantasy football
only site on the planet."
Matthew Berry, NBC Sports EDGE