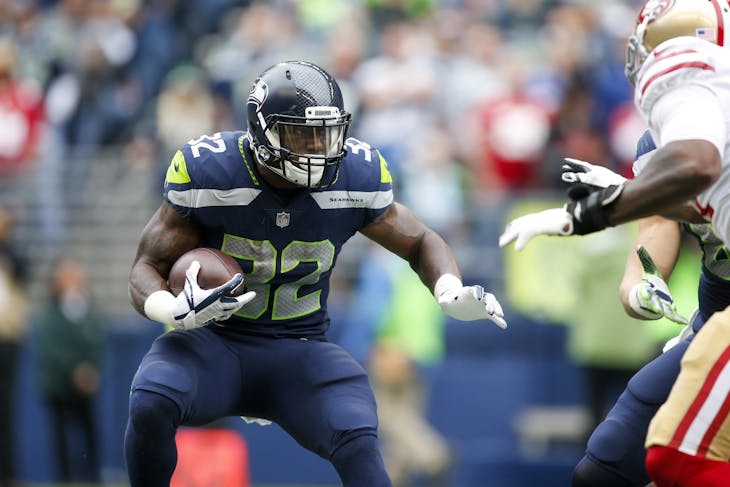
Welcome to Regression Alert, your weekly guide to using regression to predict the future with uncanny accuracy.
For those who are new to the feature, here's the deal: every week, I dive into the topic of regression to the mean. Sometimes I'll explain what it really is, why you hear so much about it, and how you can harness its power for yourself. Sometimes I'll give some practical examples of regression at work.
In weeks where I'm giving practical examples, I will select a metric to focus on. I'll rank all players in the league according to that metric, and separate the top players into Group A and the bottom players into Group B. I will verify that the players in Group A have outscored the players in Group B to that point in the season. And then I will predict that, by the magic of regression, Group B will outscore Group A going forward.
Crucially, I don't get to pick my samples (other than choosing which metric to focus on). If the metric I'm focusing on is touchdown rate, and Christian McCaffrey is one of the high outliers in touchdown rate, then Christian McCaffrey goes into Group A and may the fantasy gods show mercy on my predictions.
Most importantly, because predictions mean nothing without accountability, I track the results of my predictions over the course of the season and highlight when they prove correct and also when they prove incorrect. Here's a list of my predictions from 2020 and their final results. Here's the same list from 2019 and their final results, here's the list from 2018, and here's the list from 2017. Over four seasons, I have made 30 specific predictions and 24 of them have proven correct, a hit rate of 80%.
The Scorecard
In Week 2, I broke down what regression to the mean really is, what causes it, how we can benefit from it, and what the guiding philosophy of this column would be. No specific prediction was made.
In Week 3, I dove into the reasons why yards per carry is almost entirely noise, shared some research to that effect, and predicted that the sample of backs with lots of carries but a poor per-carry average would outrush the sample with fewer carries but more yards per carry.
In Week 4, I talked about yard-to-touchdown ratios and why they were the most powerful regression target in football that absolutely no one talks about, then predicted that touchdowns were going to follow yards going forward (but the yards wouldn't follow back).
In Week 5, we looked at ten years' worth of data to see whether early-season results better predicted rest-of-year performance than preseason ADP and we found that, while the exact details fluctuated from year to year, overall they did not. No specific prediction was made.
In Week 6, I taught a quick trick to tell how well a new statistic actually measures what you think it measures. No specific prediction was made.
In Week 7, I went over the process of finding a good statistic for regression and used team rushing vs. passing touchdowns as an example.
In Week 8, I talked about how interceptions were an unstable statistic for quarterbacks, but also for defenses.
In Week 9, we took a look at JaMarr Chase's season so far. He was outperforming his opportunities, which is not sustainable in the long term, but I offered a reminder that everyone regresses to a different mean, and the "true performance level" that Chase will trend towards over a long timeline is likely a lot higher than for most other receivers. No specific prediction was made.
In Week 10, I talked about how schedule luck in fantasy football was entirely driven by chance and, as such, should be completely random from one sample to the next. Then I checked Footballguys' staff leagues and predicted that the teams with the worst schedule luck would outperform the teams with the best schedule luck once that random element was removed from their favor.
In Week 11, I walked through how to tell the difference between regression to the mean and gambler's fallacy (which is essentially a belief in regression past the mean). No specific prediction was made.
In Week 12, I showed how to use the concept of regression to the mean to make predictions about the past and explained why the average fantasy teams were close but the average fantasy games were not. As a bonus, I threw in another quick prediction on touchdown over- and underachievers (based on yardage gained).
In Week 13, I went through the rabbit hole and investigated how performance in Regression Alert was also subject to regression to the mean, and how our current winning streak was unsustainable and destined to end sometime.
In Week 14, I talked about why larger samples were almost always better than smaller subsamples and how "hot streaks" were often just an illusion. I made two specific predictions: that a group of "hot" players would cool back down to their season average, and that the ice-cold Ja'Marr Chase would heat back up again.
In Week 15, I walked through the sad math predicting that your best fantasy team was going to lose in the playoffs.
Statistic for regression | Performance before prediction | Performance since prediction | Weeks remaining |
---|---|---|---|
Yards per Carry | Group A had 10% more rushing yards per game | Group B has 4% more rushing yards per game | None (Win!) |
Yards per Touchdown | Group A scored 9% more fantasy points per game | Group B scored 13% more fantasy points per game | None (Win!) |
Passing vs. Rushing TDs | Group A scored 42% more RUSHING TDs | Group A is scoring 33% more PASSING TDs | None (Win!) |
Defensive Interceptions | Group A had 33% more interceptions | Group B had 24% more interceptions | None (Win!) |
Schedule Luck | Group A had a 3.7% better win% | Group B has an 18.5% better win% | None (Win!) |
Yards per Touchdown | Group A scored 10% more fantasy points per game | Group B has 19% more fantasy points per game | None (Win!) |
"Hot" Players Regress | Players were performing at an elevated level | They have regressed 91.5% to their season average | 2 |
Yards per Route Run | Group A led by 109% | Group A leads by 10% | 2 |
Two weeks ago I was worried whether the Patriots 3-attempt game would tank our averages. I should have placed more faith in the process. Group B wound up cruising to another easy victory on the strength of yard-to-touchdown regression. Group B's yardage edge remained intact and Group A's touchdown edge completely vanished. At the time of the prediction, Group A was collectively averaging nearly 12 touchdowns per 1,000 yards while Group B was scoring just over 3 touchdowns per 1,000 yards. Over the last four weeks, Group A averaged 6 touchdowns per 1,000 yards while Group B scored 7.5. (Both values are within the stable "130-200 yards per touchdown" range we identified earlier, at 167 and 133, respectively.)
(I'd also like to note that excluding the Patriots game, both groups' per-game receiving yardage totals fell within 5% of their previous average. Touchdowns are unpredictable but yardage is very, very stable.)
Last week our hot players had regressed halfway back to their prior average. This week they've made it almost entirely back. There are some exceptions; Amon-Ra St. Brown averaged 8.42 points per game over the full season but 11.75 over his last four games. He scored 15.3 points in the first week of the prediction and 23.5 in the second. He's hot and only getting hotter. He's also an outlier; of the 20 remaining players (removing everyone who has missed or will miss two or more games during our sample), 18 are scoring less than they were over their "hot" streak, 16 are scoring closer to their season average than their "hot" average, and 11 are actually even below their overall season average.
As for our Ja'Marr Chase prediction... I hate making predictions with small samples because weird stuff happens in small samples. The regression overlords are apparently having some fun at my expense, because they've made the sample even smaller, still. Out of the six possible games Group A could have played, they've only appeared in one. Two games were lost to byes, two more to injuries, and one more to Covid. Right now "Group A" is still leading JaMarr Chase in yards per route run, but the overall sample sizes are minuscule and we should expect massive swings over the coming weeks still.
Finding an Edge is Only Step One
I talk a lot about sample sizes around here. It might seem like I'm borderline obsessed with them. There's a very good reason for that. Fantasy football is a game of extraordinarily slim margins; the gaps between the best and the worst GMs tend to be small.
Footballguys' staff collectively has hundreds of years of experience in this space. They've won countless awards and honors over that span. And at the end of the day, I'd venture that all of that experience and expertise results in predictions that are right maybe 55-60% of the time.
On the one hand, that's incredibly disheartening. Something that's right 60% of the time is wrong 40% of the time. You wouldn't want to trust your life to a seatbelt that had a 40% failure rate. You wouldn't want to trust your meals to a restaurant with 60% favorable reviews. You wouldn't want to trust your savings to a financial advisor who'd only bankrupted 40% of his clients.
If fantasy football only came down to a single decision each year, then a 60% success rate wouldn't cut it. But if we're able to compound our edge over and over again, suddenly the outlook is a lot rosier. Imagine I have a coin that's weighted so that it comes up heads 60% of the time. If I flip it a single time, there's a 40% chance I'll flip more tails than heads. If I flip it 9 times, those chances fall to 27%. If I flip it 99 times, those chances fall to 2%.
That's why I usually compare entire groups of players instead of individuals. The odds of one individual player from Group B outscoring one individual player from Group A are low. The odds of the entire group outscoring the entire group are high.
Similarly, that's why I run these trials for four weeks. The odds of Group A outperforming in an individual week are relatively higher than the odds of it outperforming over a four-week span. (A 16-week span would be even better, but it's not great for accountability when I just make a prediction and tell you to check back next year for the results.)
If you fade a single player because of regression, there's a great chance you'll be wrong. Selling high on Antonio Gibson because of his yards per carry average in Week 3 probably helped you a great deal. Selling "high" on Austin Ekeler might have cost you a shot at a championship. Similarly, buying low on Jonathan Taylor because of his terrible yards per carry through two weeks would have looked prescient. Buying low on Chris Carson... not so much.
If you're consistently making calls based on this information, though— if you're basing your decisions over and over and over again on 60% edges— it's virtually guaranteed that in the long run, your team will be in a better place. That's the power of small edges compounded a large number of times.
It's hard taking action knowing that it'll blow up in your face nearly (but not quite) half the time. But this key insight (tiny edges compounded ruthlessly) is the animating philosophy behind my entire approach to fantasy. I figure I'm about a 60% trader in dynasty leagues, so I trade constantly. This means that 40% of my trades blow up in my face, often spectacularly:
Looks like I'm officially winning my 4th title in 13 years in my oldest dynasty league.
— Adam Harstad (@AdamHarstad) December 23, 2019
In honor of the win, here are some of the trades I've ever made along the way.
But regression is a cruel mistress and I know if I'm going to stay ahead of her in the long haul those embarrassing losses are the price I have to pay, so I take my small advantage and I compound it and I live with the failures.
Similarly, I know that grabbing players who fall in the draft is a positive-value move. But I also know that the edge from grabbing a single faller is small, so I compound that, drafting faller after faller after faller in league after league after league. Some of my leaguemates joke that I basically let them draft my team for me. And this is true. But I trust the process and believe that the team they draft for me is more likely than not to be pretty decent.
It's not always a pretty process, but it's effective. I've been playing in Footballguys staff leagues since 2013 against players who are far better and more knowledgeable about fantasy football than I am. For the most part, my entire pre-draft preparation consists of familiarizing myself with up-to-the-minute ADP and my entire on-the-clock strategy is just "grab the guys who fall past expectations".
I expect that this process is a 55-60% advantage on any given pick, but I figure if I keep making it over and over and over again I'll come out ahead in the long run. And indeed, I've posted a lifetime 64% winning percentage against this brutal competition. (Just a 55% all-play win rate, which is a much better measure of my true performance level and which neatly aligns with my intuitions that I'm operating on something like a 5% margin.)
Sadly, I'm still searching for my first championship, but single-elimination playoffs are the ultimate small-sample trials, so I'm not sweating it. Maybe this year will be the year. (But probably not. As I noted last week, "playing the percentages over large samples" doesn't really work in the playoffs.)
This is why I place such a huge emphasis on accountability. The difference between a 60% edge compounded 99 times and a 40% edge compounded 99 times is that the first one has a 98% chance of leaving you ahead while the second has a 98% chance of leaving you broke. If you're going to compound a decision, you better be really, really, really sure it's actually an advantage. Ideally, you want something that both makes sense in theory (like regression to the mean) and holds up in practice (like regression to the mean).
But it also it helps to remind yourself that even if you're right, it's probably all for nothing, anyway. That's the maddening nature of this hobby, which rewards good decisions intermittently (at best) and seemingly bestows favor at random.
But that's also kind of what makes fantasy football so great. We do the best we can knowing full well it's likely for naught. What a great lesson on the importance of focusing on the journey rather than the destination.
The season is drawing to a close, but we have time to sneak one last prediction in under the wire. Since our sample of weeks remaining will be cut in half (2 instead of the usual 4), let's try to maximize the size of Group A and Group B to offset. And since I'm a great lover of special teams (my other gigs around here include predicting kickers and kickoff and punt returners), let's focus on the third phase.
It's commonly known that offense is more stable and predictive from one sample to the next than defense. I've written before about the dangers of playing matchups as a result. Here is where all 32 NFL franchises stand in kicker points scored and allowed per game:
Team | Points Scored | Points Allowed |
---|---|---|
Arizona Cardinals | 7.71 | 6.14 |
Atlanta Falcons | 6.29 | 6.57 |
Baltimore Ravens | 8.14 | 5.64 |
Buffalo Bills | 8.14 | 5.36 |
Carolina Panthers | 6.36 | 6.50 |
Chicago Bears | 5.86 | 7.64 |
Cincinnati Bengals | 7.79 | 6.64 |
Cleveland Browns | 5.43 | 6.21 |
Dallas Cowboys | 8.21 | 6.64 |
Denver Broncos | 6.50 | 5.50 |
Detroit Lions | 5.64 | 8.86 |
Green Bay Packers | 7.21 | 5.29 |
Houston Texans | 5.07 | 7.43 |
Indianapolis Colts | 8.14 | 4.86 |
Jacksonville Jaguars | 3.86 | 8.86 |
Kansas City Chiefs | 7.64 | 5.43 |
Las Vegas Raiders | 8.36 | 7.43 |
Los Angeles Chargers | 6.21 | 7.43 |
Los Angeles Rams | 8.86 | 6.64 |
Miami Dolphins | 5.93 | 8.00 |
Minnesota Vikings | 8.07 | 7.57 |
New England Patriots | 9.50 | 5.07 |
New Orleans Saints | 5.64 | 7.36 |
New York Giants | 6.86 | 8.21 |
New York Jets | 4.86 | 9.43 |
Philadelphia Eagles | 7.71 | 5.43 |
Pittsburgh Steelers | 7.64 | 8.50 |
San Francisco 49ers | 7.00 | 6.00 |
Seattle Seahawks | 4.71 | 6.57 |
Tampa Bay Buccaneers | 7.00 | 7.00 |
Tennessee Titans | 7.07 | 7.79 |
Washington Football Team | 6.21 | 7.64 |
I predict that (after discarding any pushes, if necessary), teams' kickers will score closer to the offense's average than the opposing defense's average in at least 55% of all remaining games.